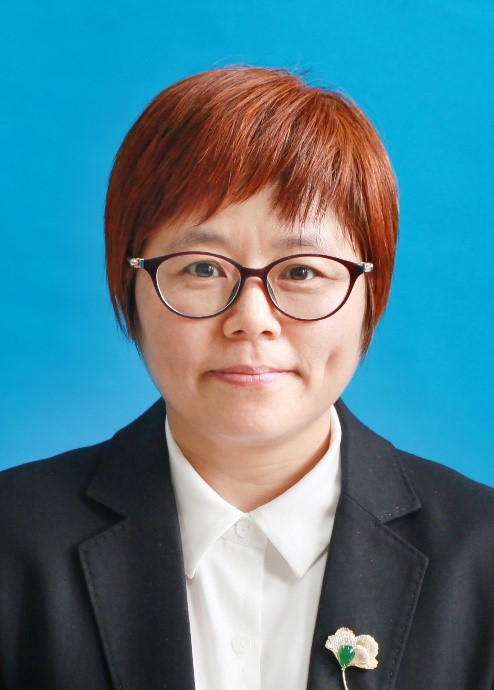 |
Prof. Dr. Haiyan Shao, University of Jinan, China
Brief: Haiyan Shao, female, PhD, associate professor, master's supervisor, teaches at the School of Mechanical Engineering, University of Jinan. Main research directions: robotics, sensor technology and application, perception measurement and control, control valves, etc. The sixth director of the Instrument Components Branch of the Chinese Instrument Society, the seventh director of the Instrument Process Branch of the Chinese Instrument Society, and the expert of the Control Valve Expert Group of the Chinese Instrument Society; selected as "ChatGPT Application Engineer (Senior)" of the Chinese Expert Think Tank Network; IEEE RCAR2019 Tutorial/Workshop Chair, IEEE ROBIO2022 Registration Chair, ISCER2024 Technical Program Member; IEEE member, CAA member, AAAS member; employed by Jinan Science and Technology Deputy General Manager, Chief Scientist of the Enterprise; Served as the head of the Control Valve Technology Research Institute jointly built by the school and the enterprise.
Scientific research: presided over the Shandong Provincial Key R&D Plan, Shandong Provincial Fund General, Shandong Provincial Small and Medium-sized Enterprises Science and Technology Innovation Project, Invention Patent Achievement Transformation Project, etc.; participated in national key special projects as a scientific research backbone. 7 invention patents and 3 utility model patents have been authorized. More than 30 SCI/EI high-level papers have been published as the first author or corresponding author.
Teaching and research: Lectures on "Testing Technology", "ROS Robot Development and Practice" and other courses. Hosted the Ministry of Education's Industry-University Cooperation and Collaborative Education Project, Shandong Province's Key Undergraduate Reform Project, Shandong Province's Graduate Case Library Construction Project, University of Jinan's Key Education Reform Project, etc. Published more than 10 teaching and research papers.
Title: Analysis and Research on the Integration of Education, Technology and Industry in Smart Education
Abstract: Under the wave of digitalization and intelligence, smart education is becoming an important force to promote educational modernization, promote educational equity and improve educational quality. This manuscript elucidates the principle, features, and current progress of intelligent learning, with a particular emphasis on the examination of digital education strategies from different nations. From multiple dimensions, the differences between traditional education and smart education are compared and analyzed. The analysis examines the specific progression of intelligent education across different nations, emphasizing the fusion of educational and technological fields, the convergence between education and the industrial sector, and the tripartite integration of industry, academia, and research, along with the challenges. The focus of this manuscript is on pioneering integration strategies through case-based teaching, the joint educational framework of industry-academic convergence, the development of a multifaceted assessment approach, and the new ‘teacher-machine-student’ teaching model. The manuscript also discusses the future development trend of smart education. Research shows that the deep integration of smart education requires multi-party collaboration, continuous innovation of teaching models, further improvement of the evaluation system, and the fostering of creative individuals equipped for the demands of forthcoming societal challenges.
|
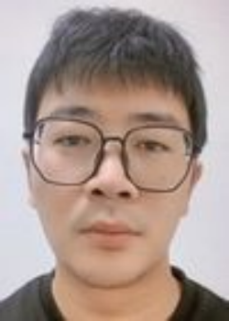 |
Dr. Lei Li, East China Normal University, China
Brief: Dr. Lei Li graduated in 2023 from East China Normal University with a degree in Data Science and Engineering. He is currently conducting postdoctoral research at the Zhejiang Lab. His research interests include natural language processing, LLM-based intelligent data analysis, data visualization, and human-computer interaction.
Title: Developing a Tool for Resolving Standard Discrepancies Using Large Language Models
Abstract: Our paper presents a useful tool leveraging Large Language Models (LLM) to resolve discrepancies between corporate and national standards. Firstly, we introduce a Food Standard Dataset comprising 1420 Chinese national standards and 100 corporate standards. Secondly, we offer a user-friendly web application to show human-readable modification suggestions. Thirdly, we propose a technique for standard information extraction, which efficiently retrieves relevant information from complete national standards. |